CAI RESEARCH
Page Under Progress

AT-A-GLANCE
This project was completed as part of the Problem-solving with People, Information, and Technology master's course at UMSI. The client for this project was the Center for Academic Innovation (CAI) and their product Michigan Online.
Timeline
3 months
Project Type
Team (5)
My Role
UX Researcher
Tools
Slack, Lucidspark, Excel, Canva, Figma
PROBLEM
Michigan Online, a learning platform developed by the University of Michigan’s Center for Academic Innovation (CAI), offers over 250 courses to help individuals develop new skills. However, navigation challenges and a lack of personalized recommendations make it difficult for users to find relevant courses efficiently, leaving them uncertain about the best options for their needs.
OUTCOME
As a team of five graduate students from UMSI, we brainstormed and researched solutions to help CAI enhance the Michigan Online platform. Our research followed six key milestones, including participant interviews, affinity wall analysis, and presenting our final report to CAI. Ultimately, this project gave me hands-on experience in client collaboration and solving tech-driven challenges as a team.
EXECUTIVE SUMMARY
BACKGROUND CONTEXT
The Center for Academic Innovation (CAI) at the University of Michigan is on a mission to revolutionize education for the future of work. A key initiative under CAI is Michigan Online, a large-scale platform offering an array of learning resources, including alternative credentials (AC), which are digital certificates or badges that signify mastery of certain skills. These are becoming increasingly important as industries evolve and technology reshapes professional landscapes. However, despite Michigan Online’s extensive library of resources, they are faced with two distinct challenges:

Navigation Challenges
The platform’s large catalog of 250+ learning experiences is difficult to filter by interest, skill level, or career goals.

Imprecise Recommendations
The existing recommendation system does not effectively cater to diverse learner goals, career pathways, or skill levels.
RESEARCH QUESTION
"How might we help users of Michigan Online make informed decisions about new learning experiences from its growing course catalog so that it can more effectively meet users' short-term and long-term needs?"
After extensive brainstorming and ideating, our team refined our H.M.W. question to focus on the need for personalized solutions that align with diverse learning goals and usage patterns. We believe that addressing this challenge would not only improve user satisfaction but also help Michigan Online better support learners in achieving their educational and professional aspirations.
PROCESS & METHODS
STAKEHOLDERS
To begin our research, we first strategized several different research methods and approaches in order to tackle our HMW question. From getting to understand our own individual strengths and skills, to brainstorming ways we can ultimately find a solution— our research to help the CAI solve one of its critical issues began with a project plan. In the initial phase, each team member conducted independent research on key topics related to our problem statement— such as trends in Gen Z job hopping. Soon after, we considered various different stakeholder groups to interview and narrowed our focus down to two main groups:

Current Students
U-M learners who are potential or active users of Michigan Online.

Design & Research Analytics Team
Responsible for building and refining the Michigan Online platform.
PRIMARY RESEARCH
We conducted a total of 5 qualitative interviews with participants based from our stakeholder groups. The interviews were structured in the following way:
Participants
4 Current U-M Students
Already enrolled at the university, with varying levels of familiarity with Michigan Online
1 CAI UX Designer
Representing the internal UX team’s perspective on platform goals and constraints
Method
30 - 40 mins. Interviews
Semi-structured with a designated interviewer and notetaker
Open-ended Questions
Allowing participants to share honest thoughts about experiences with digital learning
Focus Areas
User Goals & Motivations:
Why would a student opt to use Michigan Online?
Navigation & Discovery:
Pain points in searching or discovering courses
Platform Value:
Perceptions on the use of alternative credentials
Personal Suggestions:
What learners want to see in course previews, recommended paths, etc.
Due to time and scheduling constraints, we ended up with fewer CAI interviews than initially planned. However, the student interviews provided rich insights into platform awareness, motivation, and user behavior.
SECONDARY RESEARCH
Academic Literature
Journal Articles
Reviewed three (3) peer-reviewed journal articles highlighting global online learners’ behaviors and motivations, offering context into how Michigan Online could optimize for an international audience.
Competitive Analysis
Coursera & edX
Observed how Coursera and edX present and structure online courses, focusing on features that improve course discovery, community, and completion rates.
Artifact Analysis
CAI Blog & Social Media
Analyzed CAI’s blog to identify themes such as generative AI in education, personalized learning, and technology’s impact on engagement. Also examined CAI’s Instagram for user engagement, recurring topics, and popular themes.
RESULTS
DATA COLLECTION
All data from user interviews and secondary sources were systematically compiled into a shared spreadsheet, ensuring organization for the next steps of our process. Due to confidentiality, we used coded identification details to analyze the data. Here is a limited preview of the collected data:

AFFINITY WALL
Next, we converted our collected data into a .csv file and imported it into Lucidspark to begin constructing our affinity wall. Through affinity mapping, we clustered similar user quotes and insights, forming meta-clusters and overarching themes. This visual organization helped uncover key opportunities and design considerations for Michigan Online.


After analyzing our affinity map and reviewing the affinity wall with our peers and client, we identified three key areas in our findings that were insightful.
KEY FINDINGS
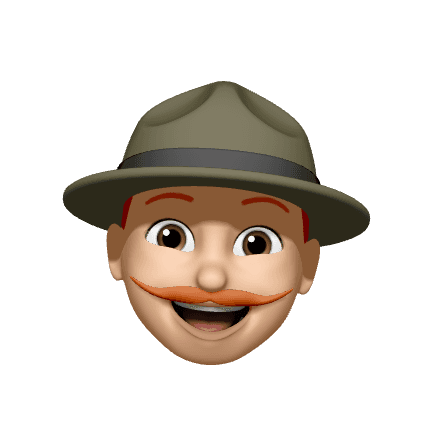
“I only found out about Michigan Online because a friend mentioned a specific course in data science. I didn’t realize it had so much more.” (PDS-05)
Low awareness among U-M students/faculty
Many U-M students either hadn’t heard of Michigan Online or considered it a supplemental resource, not a primary one. They typically discover it through accidental browsing or occasional recommendations.
Untapped Potential
There’s a missed opportunity to integrate Michigan Online courses into existing U-M coursework. Students expressed interest in how these courses could boost their academic progress or career trajectory.
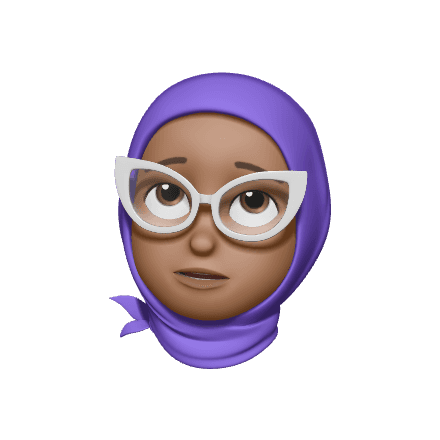
“I enroll but rarely finish. I get distracted and don’t see how it fits into my job hunt.” (PDS-04)
Low completion on Michigan Online
The platform’s 21% course completion rate indicates many users drop out. Interviewees mentioned feeling “aimless” or losing motivation after enrolling because they couldn’t connect course outcomes to specific career paths.
Competitor Insights
Coursera and Udacity, for example, highlight career mapping and skill-level filtering, helping learners pick a track aligned with their goals. They also provide robust community features to keep learners motivated.

“I typed in a broad term, but the results were either too general or not what I needed. Then I had to sort through pages of results.” (PDS-02)
Inconsistent Metadata
Users want to see course difficulty, structure (project-based vs. lecture-based), estimated completion time, and career applications up front.
Broad categories; Insufficient filtering
Students reported difficulty finding relevant courses in “umbrella” categories like “Data Science.” Subcategories, filters, or more detailed tagging are lacking.
OUTCOME
RECOMMENDATIONS
Grounded in these findings, we proposed three high-level recommendations to help Michigan Online better support its learners.
(1) Collaborate with U-M Departments
What?
Partner with key U-M departments (e.g., School of Information, College of Engineering) to integrate Michigan Online courses into the official curriculum or as recognized supplemental resources.

How?
Identify departments where course alignment is obvious (e.g., Data Science for SI students).
Work with faculty to co-develop or endorse certain courses as direct skill-gap fillers.
Create "bridge modules" or recommended pathways that connect on-campus class topics with Michigan Online’s deeper-dive offerings.
Why?
Expands the platform’s visibility and increases adoption among current students and faculty.
Aligns with CAI’s mission to serve as a leading resource for the University of Michigan.
Motivates students to see a direct connection between their on-campus coursework and additional online courses.
Potential Risks
Shifting too many resources toward U-M might reduce attention to the platform’s large international audience.
Departmental partnerships may require significant negotiation and curriculum approval processes.
(2) Provide More Career-Focused Course Information
What?
Enhance course landing pages with:
Detailed breakdown of topics and learning outcomes
Estimated time commitment
Skill prerequisites (e.g., coding background)
Real-world career applications or sample job titles
Module breakdowns (what you’ll do in Week 1, 2, 3, etc.)
How?
Conduct additional user testing or surveys to find out what top 3–5 information points are most valuable.
Populate course pages with expanded metadata.
Include “career benefits” or “applicable industries” sections, similar to competitor platforms.

Current Course Landing Page

Proposed Course Landing Page
Why?
Informs users upfront about how each course fits into their professional goals.
Addresses the “aimless” feeling, boosting motivation and completion.
"It’d be great to know how this data science course prepares me for a data analyst role, for instance." (PDS-01)
Next Steps
Validate with a broader user base (since our sample size was small).
Measure success by tracking changes in completion rates and user satisfaction post-implementation.
(3) Upgrade Search & Organization
What?
Implement enhanced filtering and sorting options (e.g., skill level, length, prerequisite knowledge).
Redesign site navigation using intuitive dropdowns and subcategories.
Tag courses with comprehensive metadata (competencies, difficulty, time, cost, etc.) to power advanced search algorithms.
How?
Adopt best practices from Coursera, Udacity, and Skillshare (organized visuals, detailed descriptions).
Introduce a "competency mapping" system letting users see current skill gaps and suggested courses to fill them.
Maintain consistent metadata standards so all courses are tagged in a uniform, user-friendly manner.

Why?
Helps users locate relevant content faster and with less frustration.
Addresses the high drop-off rate due to users’ inability to find the "right" course or losing motivation from endless scrolling.
Measuring Success
Monitor metrics such as search success rate (did a user find a relevant course quickly?), time on site, and eventual enrollment/ completion.
Aim to lift the current completion rate above 21% by making discovery more straightforward.
CONCLUSION
IMPACT
By talking to learners, reviewing CAI’s data, and studying competitor platforms, it became clear that better navigation, richer course details, and deeper connections to real career paths are essential. While Michigan Online already provides high-quality educational content, these targeted improvements will help learners discover courses that truly fit their goals and actually finish them. With an iterative, user-centered design approach, the platform can remain agile— adapting to new technologies, learner preferences, and global education demands. The insights from this project can serve as a foundation for ongoing improvements, positioning Michigan Online as a premier destination for learners eager to grow, re-skill, and thrive in tomorrow’s job market.
Next Steps
Broader UX Evaluations:
Conduct large-scale surveys or usability tests with both U-M and global learners.
Community & Mentorship Features:
Integrate discussion forums, live Q&A with instructors, or peer-to-peer learning circles to boost retention.
Language & Global Accessibility:
Add multilingual support to better serve Michigan Online’s large international audience.
AI-Driven Personalization:
Implement advanced recommendation algorithms that adapt as users progress through courses.
REFLECTION
Being part of this UX research project highlighted just how critical it is to understand user motivations— especially when course relevance to a future career can make or break engagement. By combining in-depth interviews, secondary analysis, and competitive research, we uncovered pivotal improvements to Michigan Online’s navigation and personalization. I’m excited to see how these insights can shape the platform’s future and support learners worldwide.
Thank you for reading! If you’d like to learn more about this project or see other UX research case studies, feel free to contact with me!
WORKS CITED
Contributors
Reshad Alam (reshad@umich.edu)
Yuyan Hu (yuyanhu@umich.edu)
Sureet Sarau (sureet@umich.edu)
Kyle Stocksdale (kstocks@umich.edu)
Runjin Yu (runjin@umich.edu)
References
Alam, R. (Interviewer), & Hu, Y. (Note Taker). (2024, October 28). Student Stakeholder Interview on Michigan Online platform experience [Interview]. University of Michigan. Document ID: PDS-01.
Bonk, C. J., & Zhang, K. (2006). Introducing the R2D2 model: Online learning for the diverse learners of this world. Distance education, 27(2), 249-264.
View All